How Large Language Models are Revolutionising Search
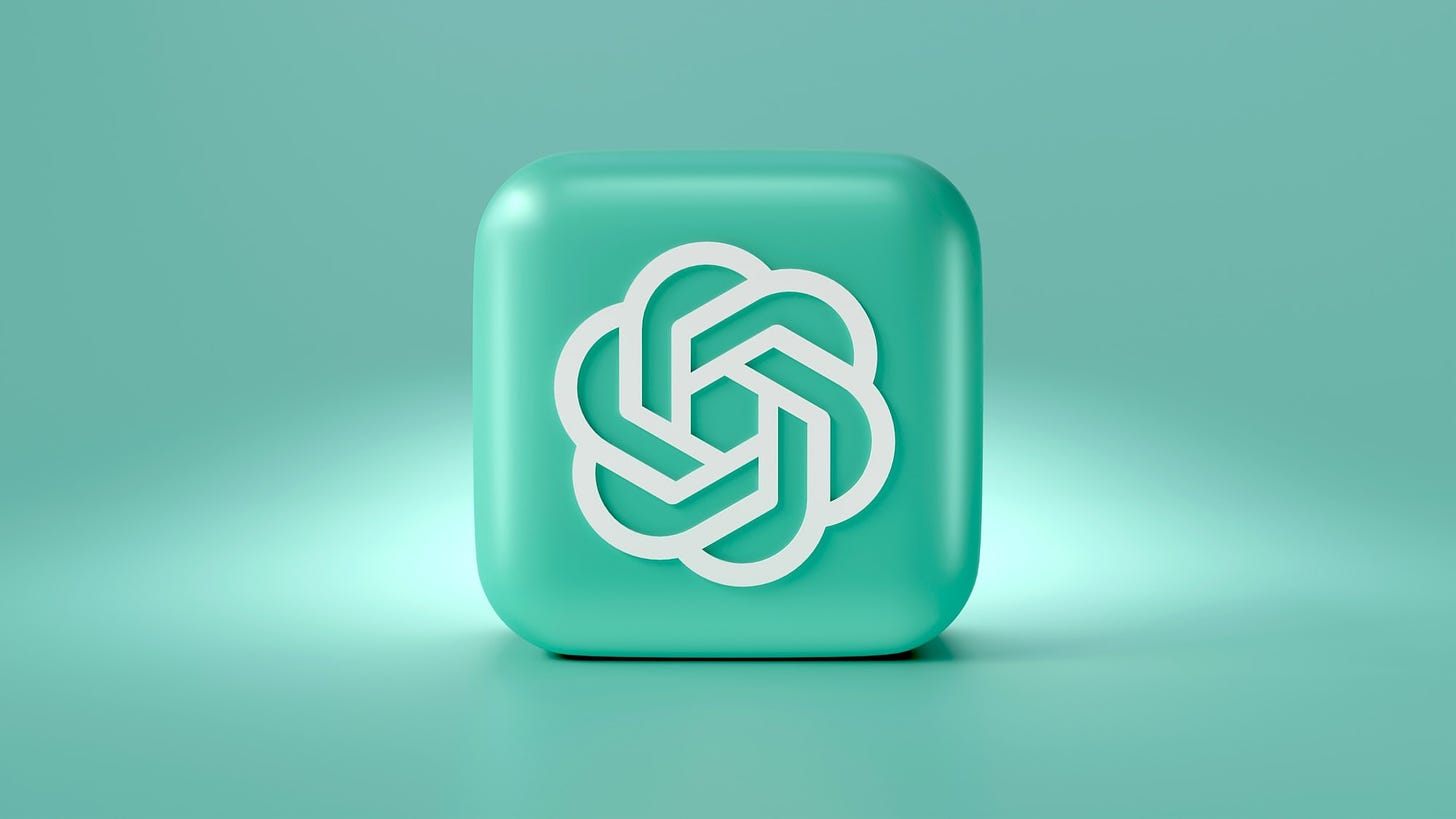
The evolution of search technology has always been tied to our pursuit of better, faster, and more accurate answers. From manually indexed directories in the early days of the web to the sophisticated algorithms of today's search engines, we've come a long way. Now, the emergence of Large Language Models (LLMs) like OpenAI's GPT series promises another leap forward, not just in the efficiency of the search process, but also in its accuracy and depth.
A Brief on LLMs
LLMs are a subset of artificial intelligence (AI) models specifically designed to understand and generate human language. These models are trained on vast amounts of text data, enabling them to produce human-like text based on the patterns they've learned. The larger these models are (in terms of parameters), the better they are at understanding context, making them potent tools for a variety of applications, from chatbots to content generation, and now, search.
Why LLMs for Search?
A recent whitepaper delves into the immense potential of LLMs in the world of search. Here are some key takeaways:
High-Quality Label Generation: One of the most resource-intensive parts of improving search algorithms is labeling. This is the process where human reviewers tag search results as relevant or irrelevant based on a query. LLMs can produce labels that are on par with human reviewers in quality, but they can do it much faster and at a fraction of the cost. This capability could significantly accelerate the iterative process of refining search algorithms.
Challenging Query Identification: Not all search queries are made equal. Some are straightforward, while others can be vague or multi-faceted. LLMs have shown an impressive knack for identifying these challenging queries. Recognizing such queries can be a valuable asset, as it allows search engines to prioritize their refinement efforts, ensuring that users get better results for complex questions.
LLMs vs. Human Participants
The potential benefits of LLMs don't stop at efficiency and cost-effectiveness. When pitted against human participants in search-related tasks, LLMs consistently perform at a comparable level, if not better in some cases. Let's consider the implications:
Consistency: Human reviewers, while highly skilled, can have off days, be influenced by biases, or make mistakes due to fatigue. LLMs, being machines, offer a level of consistency that's hard to match.
Scalability: As search engines continue to grow, dealing with the sheer volume of data becomes a challenge. LLMs can easily scale with the increasing demands, ensuring that the quality of search results doesn't diminish with growth.
Continuous Learning: While human reviewers require training sessions and refreshers, LLMs can continuously adapt and learn from new data. This ensures that they are always updated with the latest trends and nuances in language and search behavior.
A Future Powered by LLMs
Considering the benefits, it's easy to see why LLMs might be the next big thing in search. However, there are some potential concerns and challenges to consider:
Over-reliance on Machines: With the efficiency of LLMs, there's a temptation to reduce human involvement in the search refining process. While LLMs are powerful, they aren't infallible. A balanced approach, where humans and machines collaborate, might be the best way forward.
Ethical Concerns: Like all AI models, LLMs are only as good as the data they're trained on. If the training data contains biases, the LLM could perpetuate those biases in its outputs. It's essential to ensure that the training data is diverse and free from biases.
Complexity: LLMs, especially the ones with billions of parameters, are complex entities. Managing, refining, and understanding them requires expertise and resources. While they promise efficiency, they also demand investment.
Conclusion
The evolution of search is a testament to our never-ending quest for knowledge. As we stand on the cusp of another revolution powered by LLMs, it's essential to embrace the possibilities while being aware of the challenges. The future of search looks promising, with machines and humans working together to decode the vast expanse of human knowledge.